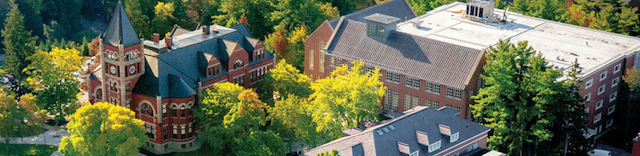
Honors Theses and Capstones
Date of Award
Spring 2024
Project Type
Senior Honors Thesis
College or School
COLSA
Department
Natural Resources
Program or Major
Environmental Science: Ecosystems
Degree Name
Bachelor of Science
First Advisor
Scott Ollinger
Second Advisor
Jack Hastings
Abstract
Leaf structural and functional traits are interrelated and can be strong predictors of leaf productivity, and it is important to understand how these traits change across both a temporal and a spatial gradient. The objective of this project is to identify and understand seasonal and height-related variations in red oak (Quercus rubra) leaf traits throughout a forest canopy, while assessing the utility of this knowledge for informing canopy models. Leaf samples were collected from red oak trees using the shotgun method and analyzed in the UNH Terrestrial Ecosystems Analysis Lab. Data were processed in Excel, JMP, and R. Leaf-level modeling and satellite imagery were used to investigate canopy reflectance at various heights throughout the forest canopy.
The key leaf traits investigated in this project are leaf mass per area (LMA), mass-based and area-based nitrogen (Nmass and Narea), and maximum photosynthetic rate (A1500mass and A1500area). LMA, A1500area, and Narea exhibited both seasonal and height-based patterns. Nmass displayed only a seasonal pattern with no discernible change across a canopy height gradient, while A1500mass displayed only a height-based trend with no seasonal pattern. This suggests that LMA is a key driver of height-based leaf trait changes, while remaining relatively uninvolved in the seasonal variability of leaf traits. Canopy reflectance was shown to vary slightly across a height gradient, with satellite imagery best aligning with upper canopy leaves. Seasonality was reflected in both modeled and satellite-based leaf reflectance. This project suggests that seasonality and height gradients are two important regulators of leaf trait changes that should be incorporated into remote sensing techniques to enhance canopy-level productivity estimates.
Recommended Citation
Kotulak, Arielle Ann, "Seasonality of Red Oak (Quercus rubra) Leaf Trait Changes Across a Canopy Height Gradient" (2024). Honors Theses and Capstones. 863.
https://scholars.unh.edu/honors/863