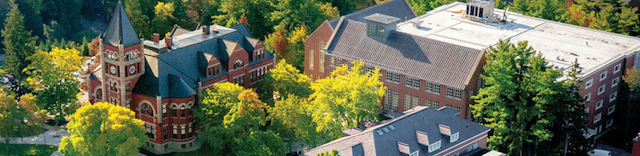
Honors Theses and Capstones
Date of Award
Spring 2024
Project Type
Senior Honors Thesis
College or School
CEPS
Department
Mathematics and Statistics
Program or Major
Statistics
Degree Name
Bachelor of Science
First Advisor
Philip Ramsey
Abstract
Addressing missing data in research is crucial for ensuring the reliability and validity of study findings, yet it remains a significant challenge. This study investigates the impact of missing data on research outcomes and explores the underutilization of existing tools for managing missingness, potentially leading to gaps in critical information with tangible implications for decision-making processes (Dziura et al.).
Focusing on the different categories of missing data—Missing Completely At Random (MCAR), Missing At Random (MAR), and Missing Not At Random (MNAR)—this research examines various imputation strategies tailored to each category. Specifically, we compare the efficacy of several model-based imputation methods, including K-Nearest Neighbors, Maximum Likelihood Estimation, and Stepwise Regression, in predicting missing values.
Through comprehensive analysis and comparison, this study aims to identify the most effective imputation approach for addressing missing data, thereby enhancing the robustness and reliability of research findings in both academic and practical contexts.
Recommended Citation
Chalumuri, Karthik, "Imputation Strategies for Different Categories of Missing Data" (2024). Honors Theses and Capstones. 793.
https://scholars.unh.edu/honors/793
Included in
Categorical Data Analysis Commons, Design of Experiments and Sample Surveys Commons, Multivariate Analysis Commons, Other Statistics and Probability Commons, Statistical Methodology Commons, Statistical Models Commons