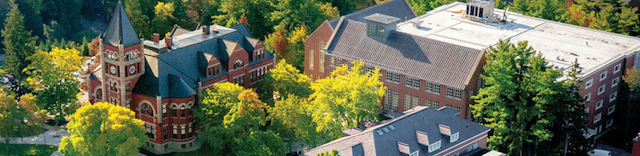
Honors Theses and Capstones
Date of Award
Spring 2023
Project Type
Senior Honors Thesis
College or School
CEPS
Department
Computer Science
Program or Major
Computer Science
Degree Name
Bachelor of Science
First Advisor
Wheeler Ruml
Abstract
Partially observable stochastic games (POSGs) are difficult domains to plan in because they feature multiple agents with potentially opposing goals, parts of the world are hidden from the agents, and some actions have random outcomes. It is infeasible to solve a large POSG optimally. While it may be tempting to design a specialized algorithm for finding suboptimal solutions to a particular POSG, general-purpose planning algorithms can work just as well, but with less complexity and domain knowledge required. I explore this idea in two different POSGs: Navy Defense and Duelyst.
In Navy Defense, I show that a specialized algorithm framework, goal-driven autonomy, which requires a complex subsystem separate from the planner for explicitly reasoning about goals, is unnecessary, as simple general planners such as hindsight optimization exhibit implicit goal reasoning and have strong performance.
In Duelyst, I show that a specialized expert-rule-based AI can be consistently beaten by a simple general planner using only a small amount of domain knowledge. I also introduce a modification to Monte Carlo tree search that increases performance when rollouts are slow and there are time constraints on planning.
Recommended Citation
McKenney, Bryan, "General-Purpose Planning Algorithms In Partially-Observable Stochastic Games" (2023). Honors Theses and Capstones. 773.
https://scholars.unh.edu/honors/773