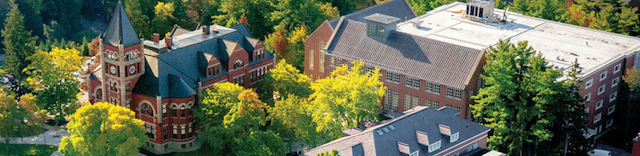
Honors Theses and Capstones
Date of Award
Spring 2022
Project Type
Senior Honors Thesis
College or School
CEPS
Department
Mathematics and Statistics
Program or Major
Statistics
Degree Name
Bachelor of Science
First Advisor
Easton White
Second Advisor
Philip Ramsey
Abstract
COVID-19 caused state and nation-wide lockdowns, which altered human foot traffic, especially in restaurants. The seafood sector in particular suffered greatly as there was an increase in illegal fishing, it is made up of perishable goods, it is seasonal in some places, and imports and exports were slowed. Foot traffic data is useful for business owners to have to know how much to order, how many employees to schedule, etc. One issue is that the data is very expensive, hard to get, and not available until months after it is recorded. Our goal is to not only find covariates that can accurately predict foot traffic in United States seafood restaurants, but find ones that are free, easily accessible, and available in real time.
We built multiple models in R-Studio using cross validation and compared using mean squared error. We found that the google search trend of ‘seafood takeout’ was most strongly correlated to foot traffic, correlation coefficient is .602, and appeared in every model. The model with the lowest mean squared error is a generalized linear model (GLM) with google search trends, unemployment rate, and average covid cases per week as the predictors. These predictors were selected using backwards selection by akaike information criterion (AIC). We are still awaiting new foot traffic data to test our model on, which shows how long it takes to receive the data. Next steps would be to look at if more models can be made that focus on individual states as well as finding other covariates that could be used.
Recommended Citation
Beaulieu, Isabel Paige, "Finding the Best Predictors for Foot Traffic in US Seafood Restaurants" (2022). Honors Theses and Capstones. 635.
https://scholars.unh.edu/honors/635
Included in
Analysis Commons, Applied Statistics Commons, Aquaculture and Fisheries Commons, Biostatistics Commons, Data Science Commons, Management Sciences and Quantitative Methods Commons, Other Food Science Commons, Statistical Models Commons